About the Workshop
In the ever-evolving landscape of information retrieval, harnessing the power of graph-based approaches has emerged as a transformative force. Graph theory, which has long been among the backbones of computer science and data analysis, is becoming crucial within personalization and search domains, especially with the recent widespread research on Knowledge Graphs and Graph Neural Networks. These models offer a versatile set of techniques and tools that enhance information retrieval's effectiveness and relevance. They leverage the power of interconnected data to provide users with more accurate, context-aware, and personalized search results and recommendations.
The First International Workshop on Graph-Based Approaches in Information Retrieval (IRonGraphs 2024) serves as a pivotal venue where researchers, practitioners and enthusiasts from diverse backgrounds converge to explore and discuss the integration of graph-based methodologies into information retrieval studies and applications.
Topics
The workshop welcomes contributions on all topics related to graph-based approaches in Information Retrieval, focused (but not limited) to:
Graph-Based Models for Information Retrieval
Surveys on various graph-based models, including Graph Neural Networks, and their applications in Information Retrieval.
Graph-Based Ranking Methods
Exploration of algorithms that leverage graphs to improve document ranking and relevance in search engines.
Personalised Search and Recommendation
How graph-based approaches enable personalised search and recommendation through graphs.
Knowledge Graphs and Information Retrieval
The use of knowledge graphs to enhance search results, answer complex queries, and provide explanations.
Graph-Based Query Expansion
Techniques for expanding user queries using graph-based methods such that recall and precision in search are improved.
Semantic Understanding
How graph-based models capture semantic relationships between entities and concepts to understand user intent.
Behavioural User Profiling
How behavioural user profiling through graphs impacts IR, enabling more context-aware and personalised search.
Cross-Domain and Cross-Lingual Retrieval
Strategies that adopt graphs to facilitate effective cross-domain and cross-lingual information retrieval.
Evaluation Metrics and Benchmarks
Evaluation metrics and benchmark datasets for assessing the performance of graph-based approaches in Information Retrieval.
Fairness, Explainability, and Privacy
Ethical implications related to the use of graph-based methods in Information Retrieval.
Real-World Applications
Case studies and real-world applications of graph-based techniques (e.g. in education, healthcare, and tourism).
Future Directions and Challenges
Emerging trends, open research challenges, and opportunities for further innovation of graph-based information retrieval.
Call for Papers
Submission details
We invite authors to submit unpublished original papers, written in English. Submitted papers should not have been previously published or accepted for publication in substantially similar form in any peer-reviewed venue, such as journals, conferences, or workshops.
The authors should consult the Springer's authors' guidelines and use their proceedings templates, either LaTeX (including Overleaf) or Word.
Papers should be submitted as PDF files to Easychair at https://easychair.org/conferences/?conf=irongraphs2024.
We will consider three different submission types:
- Full papers (12 pages) should be clearly placed with respect to the state of the art and state the contribution of the proposal in the domain of application. In particular, research papers should describe the methodology in detail, experiments should be repeatable, and a comparison with the existing approaches in the literature should be made.
- Reproducibility papers (12 pages) should repeat prior experiments using the original source code and datasets to show how, why, and when the methods work or not (replicability papers) or should repeat prior experiments, preferably using the original source code, in new contexts (e.g., different domains and datasets, different evaluation and metrics) to further generalize and validate or not previous work (reproducibility papers).
- Short papers (6 pages) should present novel, though-provoking ideas and addressing innovative application areas within the workshop topics, with comparable experimental results, even if preliminary.
- Position papers (4 pages) should introduce new point of views in the workshop topics or summarize the experience of a group in the field.
Submissions may exceed the indicated number of pages only with references.
All submissions will go through a double-blind review process and be reviewed by at least three reviewers on the basis of relevance for the workshop, novelty/originality, significance, technical quality and correctness, quality and clarity of presentation, quality of references and reproducibility.
Submitted papers will be rejected without review in case they are not properly anonymized, do not comply with the template, or do not follow the above guidelines.
The accepted papers and the material generated during the meeting will be available on the workshop website. The workshop proceedings are intended to be published as a Springer's Communications in Computer and Information Science (CCIS) revised post-proceedings volume, indexed on Google Scholar, DBLP and Scopus. The authors of selected papers may be invited to submit an extended version in a journal special issue.
Please be aware that at least one author per paper needs to register and attend the workshop to present the work.
We expect authors, the program committee, and the organizing committee to adhere to the ACM's Conflict of Interest Policy and the ACM's Code of Ethics and Professional Conduct.
Important dates
- Submission:
January 15, 2024January 22, 2024 (EXTENDED) - Author notification: February 19, 2024
- Camera-ready: February 26, 2024
- Workshop day: March 24, 2024
All the deadlines are set at 11:59 PM AoE.
Keynote
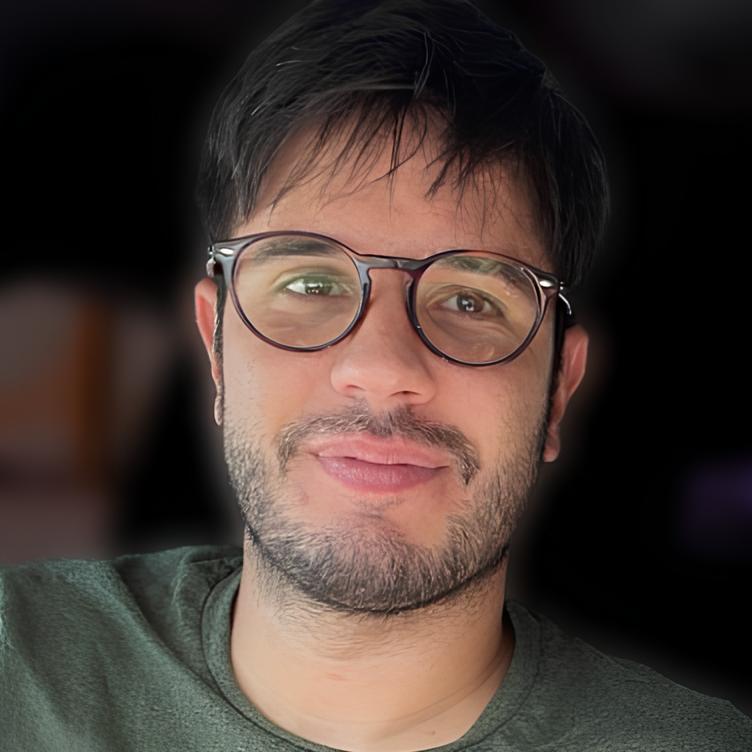
Francesco Fabbri
Spotify, Spain
Graph Foundation Model for Personalization
Abstract: In the ever-evolving landscape of digital audio content, integrating diverse information sources such as consumption signals and content-based representations is becoming increasingly critical for building state-of-the-art solutions. For these reasons, designing a foundation model that could tailor item recommendations or search results to individual users can be challenging. In this talk, we take a step towards defining a Graph Foundation Model (GFM) for Personalization. To do so, we demonstrate the importance of graph-based learning methods for personalization at scale. Specifically, our findings illustrate how graph learning in combination with large language models can address multiple downstream tasks within a graph foundational architecture (GFM) in the domain of talk audio space, including podcast and audiobook personalization.
Bio: Dr Francesco Fabbri is a Research Scientist at Spotify, working on Representation Learning and Generative Responsible AI for personalization. He earned his Ph.D. in Computer Science (cum laude) from Pompeu Fabra University, Barcelona, focusing on analyzing and mitigating unintended effects in online social platforms. His research has been published in top-tier conferences including CIKM, theWebConf, ICWSM, and ECIR. Notable awards include the Best Paper Award at theWebConf and the Ted Nelson Award at Hypertext. Francesco obtained a Master’s Degree (Honours) in Data Science and a Bachelor of Science in Statistics both from Sapienza, University of Rome.
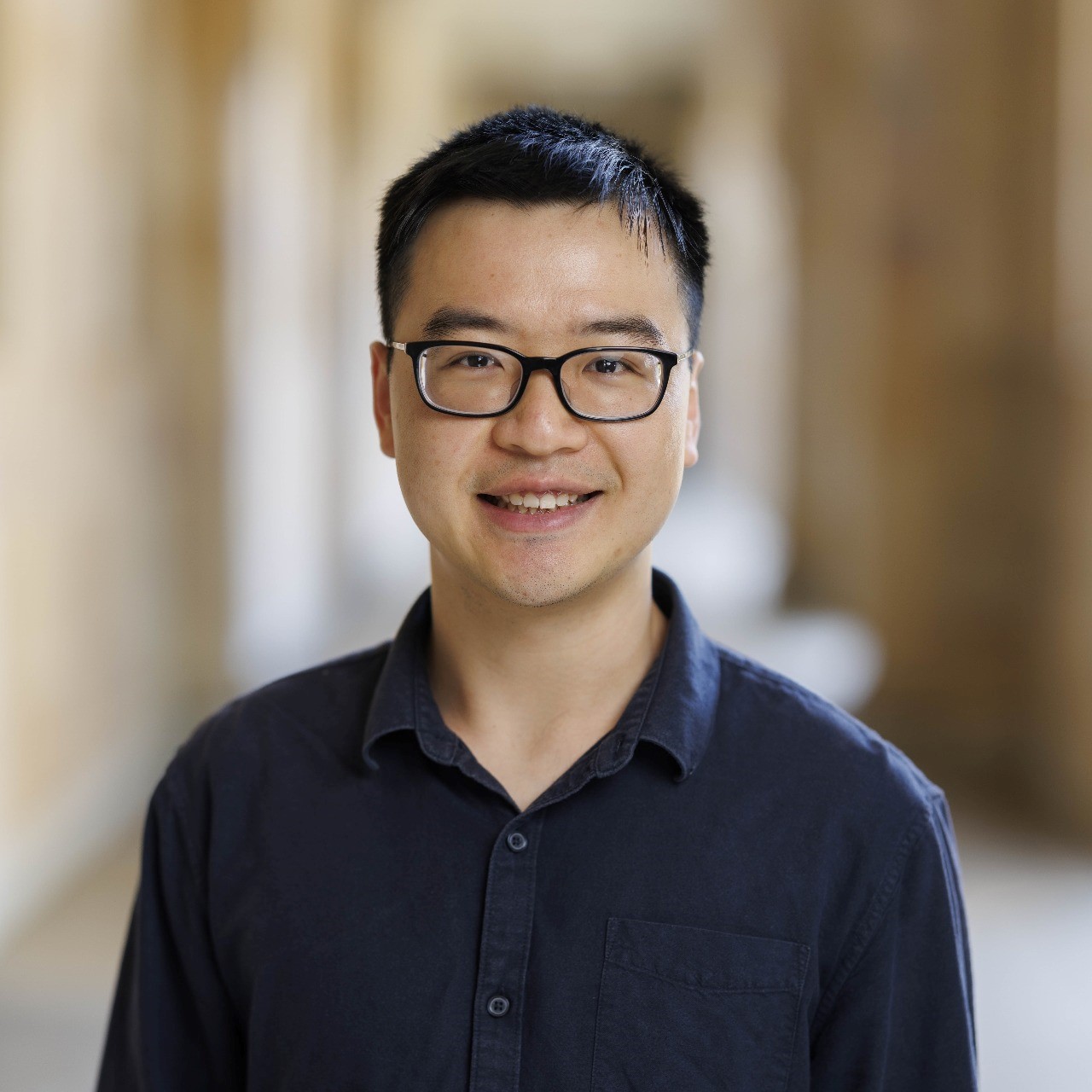
Ruihong Qiu
University of Queensland, Australia
Graph Learning Methods in Session-based Recommendations and Legal Case Retrieval
Abstract: Graph learning has emerged as a powerful technique for capturing the structural characteristics of various scenarios within the realm of information retrieval. This seminar will delve into two illustrative examples: session-based recommendations and legal case retrieval. In session-based recommendations, user history is converted into a graph, allowing for the integration of structural insights into user behaviour. Similarly, in legal case retrieval, lengthy legal cases can be transformed into graphs, facilitating the preservation of legal context across different parties involved. In essence, when the fundamental unit (such as user, item, query, document, etc.) in information retrieval problems possesses significant structural features, leveraging graph data proves to be a promising approach for harnessing valuable information.
Bio: Dr Ruihong Qiu is a Postdoctoral Research Fellow in the School of Electrical Engineering and Computer Science, the University of Queensland (UQ). He obtained his PhD degree in Computer Science at UQ in 2022, where he works on effective modelling of sequential patterns of user behaviour in recommender systems. Ruihong’s research is mainly focused on data science methods for sequential and graph data on modern online platforms and is published at major venues of information retrieval and knowledge discovery, such as SIGIR, WSDM, ICDM, MM, ICCV and TOIS. His research has won the Best Paper Award at ADC 2023, and the International Competition of Social Media Mining for Health 2023.
Program
Sunday, March 24, 2024
Welcome
Keynote: Graph Foundation Model for Personalization
Francesco Fabbri, Research Scientist at Spotify (Spain)
Coffee Break
Paper Session (15min Talk + 5min Q&A)
-
KGUF: Simple Knowledge-aware Graph-based Recommender with User-based Semantic Features Filtering
Salvatore Bufi; Alberto Carlo Maria Mancino; Antonio Ferrara; Daniele Malitesta; Tommaso Di Noia; Eugenio Di Sciascio
-
The Impact of Source-Target Node Distance on Vicious Adversarial Attacks in Social Network Recommendation Systems
Federico Albanese; Giovanni Trappolini; Lorenzo Scarlino; Fabrizio Silvestri
-
The Effectiveness of Graph Contrastive Learning on Mathematical Information Retrieval
Pei Syuan Wang; Hung-Hsuan Chen
-
Identifying User Shopping Needs in Voice Product Questions for Proactive Shopping Recommendations
Besnik Fetahu; Nachshon Cohen; Elad Haramaty; Liane Lewin-Eytan; Oleg Rokhlenko; Shervin Malmasi
Salvatore Bufi; Alberto Carlo Maria Mancino; Antonio Ferrara; Daniele Malitesta; Tommaso Di Noia; Eugenio Di Sciascio
Federico Albanese; Giovanni Trappolini; Lorenzo Scarlino; Fabrizio Silvestri
Pei Syuan Wang; Hung-Hsuan Chen
Besnik Fetahu; Nachshon Cohen; Elad Haramaty; Liane Lewin-Eytan; Oleg Rokhlenko; Shervin Malmasi
Lunch Break
Spotlight Session (5min Talk + 5min Q&A)
-
CaseGNN: Graph Neural Networks for Legal Case Retrieval with Text-Attributed Graphs
Yanran Tang; Ruihong Qiu; Yilun Liu; Xue Li; Zi Huang
-
XSearchKG: A Platform for Explainable Keyword Search over Knowledge Graphs
Leila Feddoul; Martin Birke; Sirko Schindler
-
Performance Comparison of Session-Based Recommendation Algorithms Based on GNNs
Faisal Shehzad; Dietmar Jannach
-
Event-specific Document Ranking through Multi-stage Query Expansion Using Knowledge Graphs
Sara Abdollahi; Tin Kuculo; Simon Gottschalk
-
Hypergraphs with Attention on Reviews for Explainable Recommendation
Theis E. Jendal; Trung-Hoang Le; Hady Lauw; Matteo Lissandrini; Peter Dolog; Katja Hose
-
Overcoming Recall Failures in Ad Hoc Search using Graphs
Sean MacAvaney
Yanran Tang; Ruihong Qiu; Yilun Liu; Xue Li; Zi Huang
Leila Feddoul; Martin Birke; Sirko Schindler
Faisal Shehzad; Dietmar Jannach
Sara Abdollahi; Tin Kuculo; Simon Gottschalk
Theis E. Jendal; Trung-Hoang Le; Hady Lauw; Matteo Lissandrini; Peter Dolog; Katja Hose
Sean MacAvaney
Poster Session
Coffee Break
Keynote: Graph Learning Methods in Session-based Recommendations and Legal Case Retrieval
Ruihong Qiu, Associate Lecturer and a Postdoctoral Research Fellow at The University of Queensland (Australia)
Final Discussion and Closing Remarks
Proceedings
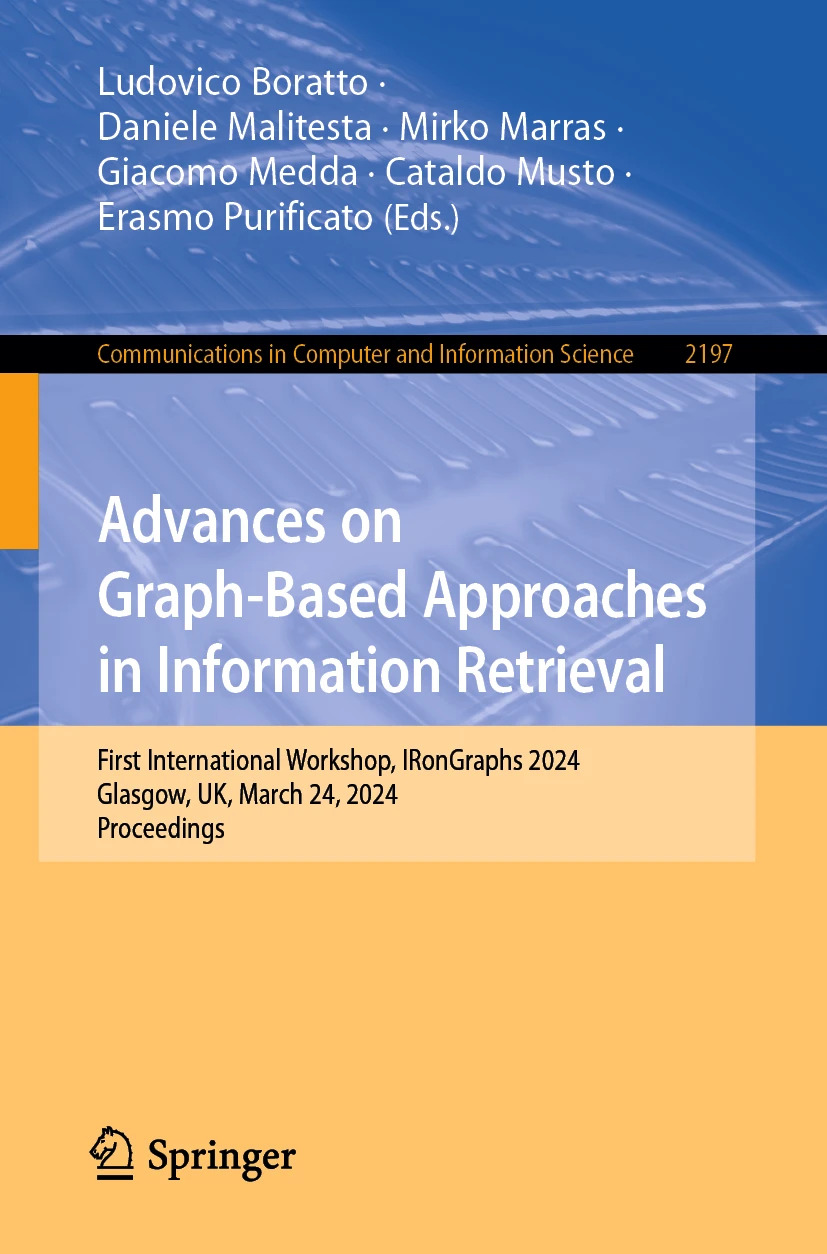
Advances on Graph-Based Approaches in Information Retrieval
Proceedings of the First International Workshop, IRonGraphs 2024, Selected Revised Papers
Click here to get free access rights to the proceedings on the Springer website
Organization
Workshop Chairs
Program Committee
* The list of PC members will be constantly updated- Marcelo Armentano - UNICEN, Argentina
- Giacomo Balloccu - University of Cagliari, Italy
- Alejandro Bellogin - Universidad Autonoma de Madrid, Spain
- Salvatore Bufi - Polytechnic University of Bari, Italy
- Jeongwhan Choi - Yonsei University, South Korea
- Edoardo D'Amico - University College Dublin, Ireland
- Danilo Dessì - GESIS, Leibniz Institute for the Social Sciences, Germany
- Claudio Di Sipio - University of L'Aquila, Italy
- Manuel Dileo - University of Milan, Italy
- Maurizio Ferrari Dacrema - Polytechnic University of Milan, Italy
- Rima Hazra - Singapore University of Technology and Design, Singapore
- Alireza Javadian Sabet - University of Pittsburgh, USA
- Dominik Kowald - Know-Center, Graz University of Technology, Austria
- Emanuel Lacic - Infobip
- Alberto Carlo Maria Mancino - Polytechnic University of Bari, Italy
- Tendai Mukande - Dublin City University, Ireland
- Julia Neidhardt - TU Wien, Austria
- Linsey Pang - Salesforce
- Ladislav Peska - Charles University, Prague, Czechia
- Claudio Pomo - Polytechnic University of Bari, Italy
- Vaijanath Rao - Quicken Inc.
- Mukul Singh - Microsoft
- Tokala Yaswanth Sri Sai Santosh - IIT Kharagpur, India
- Antonela Tommasel - Aarhus University, Denmark & CONICET-UNCPBA, ISISTAN Research Institute, Argentina
- Eva Zangerle - University of Innsbruck, Austria
Registration
Registration to the workshop will be managed by the ECIR 2024 organization.
Contacts
For any request you might have, please contact irongraphsworkshop@gmail.com.
You can also follow us on X (ex Twitter): @IRonGraphsWS.